Computer Science Department Thesis Defense - Ahmed Aboulfotouh
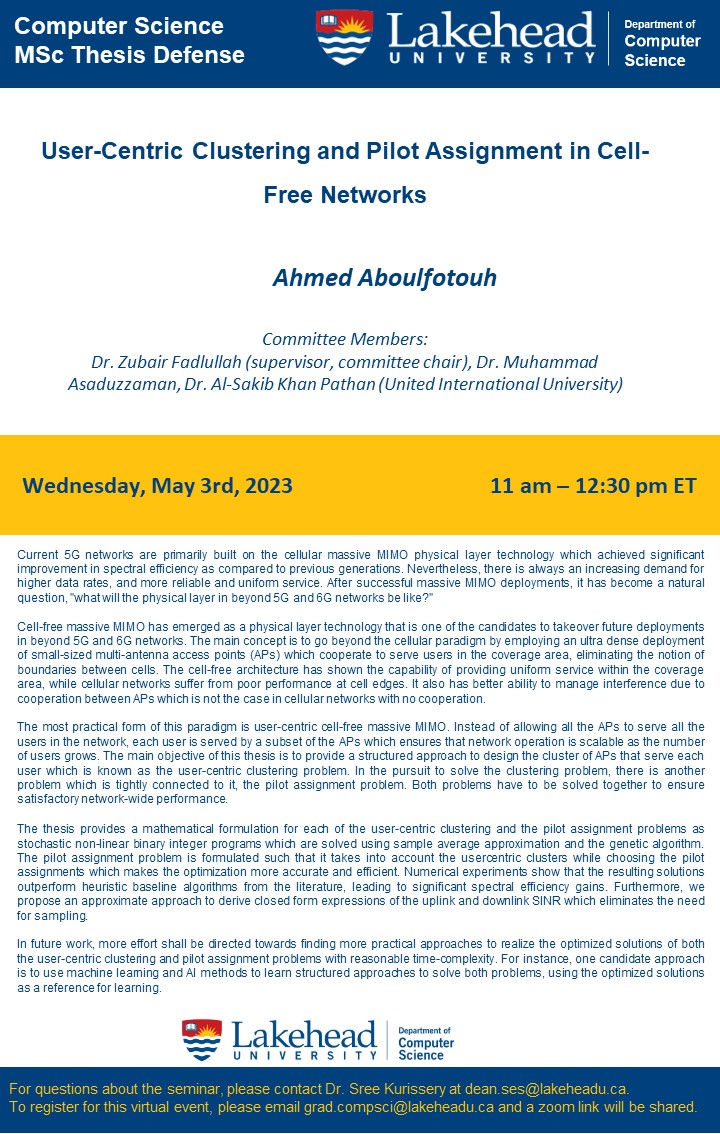
Please join the Computer Science Department for the upcoming thesis defense:
Presenter: Ahmed Aboulfotouh
Thesis title: User-Centric Clustering and Pilot Assignment in Cell-Free Networks
Abstract: Current 5G networks are primarily built on the cellular massive MIMO physical layer technology which achieved significant improvement in spectral efficiency as compared to previous generations. Nevertheless, there is always an increasing demand for higher data rates, and more reliable and uniform service. After successful massive MIMO deployments, it has become a natural question, "what will the physical layer in beyond 5G and 6G networks be like?"
Cell-free massive MIMO has emerged as a physical layer technology that is one of the candidates to takeover future deployments in beyond 5G and 6G networks. The main concept is to go beyond the cellular paradigm by employing an ultra dense deployment of small-sized multi-antenna access points (APs) which cooperate to serve users in the coverage area, eliminating the notion of boundaries between cells. The cell-free architecture has shown the capability of providing uniform service within the coverage area, while cellular networks suffer from poor performance at cell edges. It also has better ability to manage interference due to cooperation between APs which is not the case in cellular networks with no cooperation.
The most practical form of this paradigm is user-centric cell-free massive MIMO. Instead of allowing all the APs to serve all the users in the network, each user is served by a subset of the APs which ensures that network operation is scalable as the number of users grows. The main objective of this thesis is to provide a structured approach to design the cluster of APs that serve each user which is known as the user-centric clustering problem. In the pursuit to solve the clustering problem, there is another problem which is tightly connected to it, the pilot assignment problem. Both problems have to be solved together to ensure satisfactory network-wide performance.
The thesis provides a mathematical formulation for each of the user-centric clustering and the pilot assignment problems as stochastic non-linear binary integer programs which are solved using sample average approximation and the genetic algorithm. The pilot assignment problem is formulated such that it takes into account the usercentric clusters while choosing the pilot assignments which makes the optimization more accurate and efficient. Numerical experiments show that the resulting solutions outperform heuristic baseline algorithms from the literature, leading to significant spectral efficiency gains. Furthermore, we propose an approximate approach to derive closed form expressions of the uplink and downlink SINR which eliminates the need for sampling.
In future work, more effort shall be directed towards finding more practical approaches to realize the optimized solutions of both the user-centric clustering and pilot assignment problems with reasonable time-complexity. For instance, one candidate approach is to use machine learning and AI methods to learn structured approaches to solve both problems, using the optimized solutions as a reference for learning.
Committee Members:
Dr. Zubair Fadlullah (supervisor, committee chair), Dr. Muhammad Asaduzzaman, Dr. Al-Sakib Khan Pathan (United International University)
Please contact grad.compsci@lakeheadu.ca for the Zoom link.
Everyone is welcome.