Research in Action: Ultra-sensitive sensors signal a bright future in at-home health care
Published in The Chronicle Journal, Thursday, July 7th, 2022
BY JULIO HELENO GOMES
One of the most promising developments in the medical field centres on tiny sensors that can potentially provide the user with instant information on their health. In terms of heart monitoring, recent advancements in wearable devices are bringing more and more information into the hands of its user.
However, one of the challenges in accessing data from these devices is background noise. But work undertaken by Lakehead University researchers is pointing a way around this problem. By using deep learning-based data-driven models, Dr. Zubair Fadlullah says they have a way to potentially overcome the issue of low frequency noise.
“I would say it’s a very significant achievement,” says Fadlullah, an associate professor in the department of Computer Science. “For the people who fabricate, who manufacture these sensors, it will be a breakthrough for them.”
Fadlullah, who came to Lakehead in 2019 from Japan, has a background in information sciences. His research focuses on wireless communication systems, the Internet of Things, and smart health technology. As joint Lakehead University-Thunder Bay Regional Health Research Institute Research Chair, his interest is medical analytics and biomedical computing.
In particular, he is looking at the “resource constraint” of computing devices. These sensors and other biomedical equipment don’t have “local intelligence,” meaning they only monitor and collect data and upload it to be processed and then sent back to the user, for example, a doctor, who will make use of that analysis. “Resource constraint” usually refers to battery power and means the sensor doesn’t integrate with the computer.
“That’s why the sensor collects the data, sends the data out to some remote server -- the Internet or the cloud -- and the cloud does the processing and analysis, gives some result, and then transmits the result back to the owner,” Fadlullah explains. “These conventional sensors typically work like dumb monitors. They are non-intelligent.”
One example is the electrocardiogram (ECG or EKG), which checks heart function by measuring electrical activity. A well-trained technician can interpret the signals to determine if a person has arrhythmia, coronary heart disease or other conditions that require attention. Generally, an ECG involves attaching small, sticky sensors called electrodes to the arms, legs and chest.
“This is intrusive and requires the patient to go to a clinical setting,” Fadlullah notes.
There are now many types of wearable devices that passively monitor heart activity. But they do not offer clinical-grade ECG readings.
“We want to create non-intrusive ECG monitoring without putting additional burden on the user – without them having to put these electrodes on their body or wear some kind of watch all the time that can perform continuous heart monitoring,” he says.
The other issue is the device that monitors the magnetic field that arises from electrical activity of the heart (MCG or magnetocardiography) is large, sits in a magnetically shielded room and requires cooling. The goal is to develop technology that measures electrical activity of the heart in a more compact package, specifically small magnetic sensors.
“If we can do that, we have the potential of generating lots of these sensors, placing them in the residence of users, in hospitals, in clinics,” Fadlullah says. “With such proof-of-concept, patients don’t have to hold these electrodes on their body, they don’t have to keep the wearable devices on all the time. It just makes things much easier for them, to have this non-intrusive cardiac reading.”
The problem is that there are so many magnetic fields around that they create low frequency noise. Working with collaborators at Tohoku University in Japan, Fadlullah and his research assistant used machine learning to combine two AI architectures targeted for magnetic sensors to denoise or clean up this interference.
“The main benefit of this research is that it can pave the way for the future of non-invasive long-term patient monitoring in remote settings,” says Sadman Sakib, the graduate assistant on this project.
Sakib, who graduated in 2021 with a Master in Computer Science degree and is now working as a data scientist in Kingston, Ont., believes this research can expand to other areas of medicine, providing faster access to health services, especially in rural and remote communities.
Fadlullah, along with his students and research collaborators, has published several papers to show machine learning-based models can be used to overcome the obstacle associated with specific magnetic sensors in cardiac monitoring.
“Having this kind of small and ultra-sensitive sensor is all good. But if they couldn’t solve the challenge of filtering out these low frequency noises, these sensors wouldn’t be very useful, wouldn’t be very practical,” he says. “Solving this problem of filtering out the low frequency has opened up so many applications for our collaborators. I believe this is almost a breakthrough.”
In the meantime, he has extended the theoretical part of the work to study early warning sensing of cardiac activity. The study involves graduate assistant Mohamed Elshafei and is supported by the Qatar National Research Fund.
Research in Action highlights the work of Lakehead University in various fields of research.
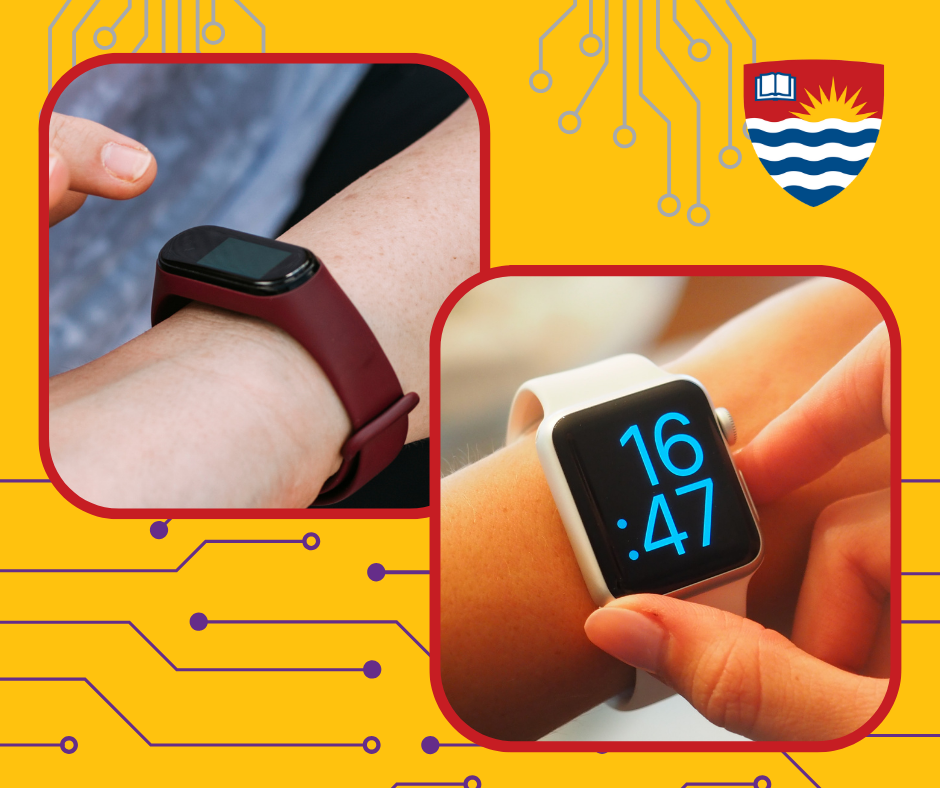