Computer Science Department Thesis Defense - Udhaya Kumar Rajendran
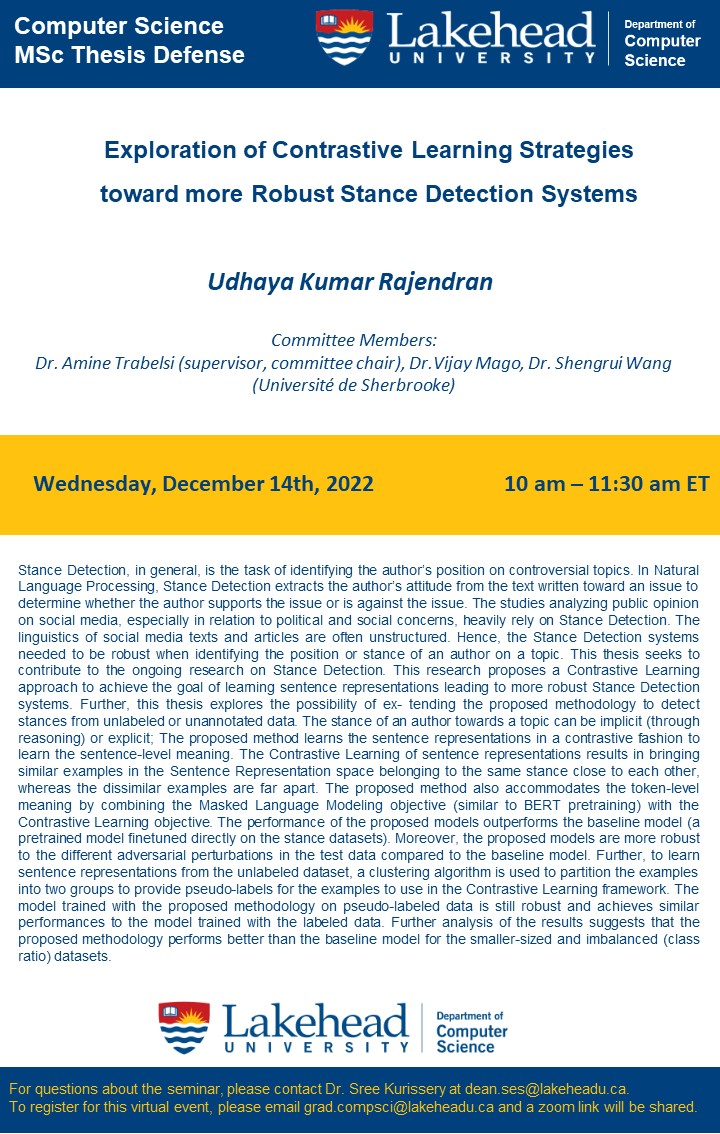
Please join the Computer Science Department for the upcoming thesis defense:
Presenter: Udhaya Kumar Rajendran
Thesis title: Exploration of Contrastive Learning Strategies toward more Robust Stance Detection Systems
Abstract: Stance Detection, in general, is the task of identifying the author’s position on controversial topics. In Natural Language Processing, Stance Detection extracts the author’s attitude from the text written toward an issue to determine whether the author supports the issue or is against the issue. The studies analyzing public opinion on social media, especially in relation to political and social concerns, heavily rely on Stance Detection. The linguistics of social media texts and articles are often unstructured. Hence, the Stance Detection systems needed to be robust when identifying the position or stance of an author on a topic. This thesis seeks to contribute to the ongoing research on Stance Detection. This research proposes a Contrastive Learning approach to achieve the goal of learning sentence representations leading to more robust Stance Detection systems. Further, this thesis explores the possibility of ex- tending the proposed methodology to detect stances from unlabeled or unannotated data. The stance of an author towards a topic can be implicit (through reasoning) or explicit; The proposed method learns the sentence representations in a contrastive fashion to learn the sentence-level meaning. The Contrastive Learning of sentence representations results in bringing similar examples in the Sentence Representation space belonging to the same stance close to each other, whereas the dissimilar examples are far apart. The proposed method also accommodates the token-level meaning by combining the Masked Language Modeling objective (similar to BERT pretraining) with the Contrastive Learning objective. The performance of the proposed models outperforms the baseline model (a pretrained model finetuned directly on the stance datasets). Moreover, the proposed models are more robust to the different adversarial perturbations in the test data compared to the baseline model. Further, to learn sentence representations from the unlabeled dataset, a clustering algorithm is used to partition the examples into two groups to provide pseudo-labels for the examples to use in the Contrastive Learning framework. The model trained with the proposed methodology on pseudo-labeled data is still robust and achieves similar performances to the model trained with the labeled data. Further analysis of the results suggests that the proposed methodology performs better than the baseline model for the smaller-sized and imbalanced (class ratio) datasets.
Committee Members:
Dr. Amine Trabelsi (supervisor, committee chair), Dr.Vijay Mago, Dr. Shengrui Wang (Université de Sherbrooke)
Please contact grad.compsci@lakeheadu.ca for the Zoom link.
Everyone is welcome.