Computer Science Department Thesis Defense - Atharva Phatak
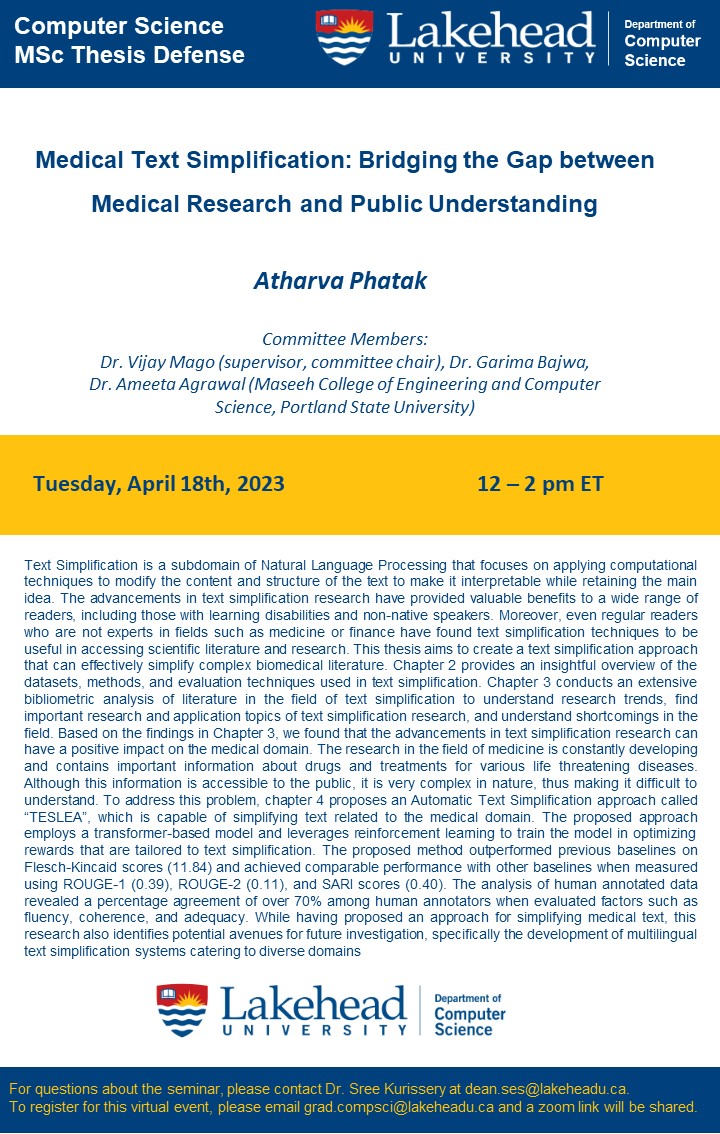
Please join the Computer Science Department for the upcoming thesis defense:
Presenter: Atharva Phatak
Thesis title: Medical Text Simplification: Bridging the Gap between Medical Research and Public Understanding
Abstract: Text Simplification is a subdomain of Natural Language Processing that focuses on applying computational techniques to modify the content and structure of the text to make it interpretable while retaining the main idea. The advancements in text simplification research have provided valuable benefits to a wide range of readers, including those with learning disabilities and non-native speakers. Moreover, even regular readers who are not experts in fields such as medicine or finance have found text simplification techniques to be useful in accessing scientific literature and research. This thesis aims to create a text simplification approach that can effectively simplify complex biomedical literature. Chapter 2 provides an insightful overview of the datasets, methods, and evaluation techniques used in text simplification. Chapter 3 conducts an extensive bibliometric analysis of literature in the field of text simplification to understand research trends, find important research and application topics of text simplification research, and understand shortcomings in the field. Based on the findings in Chapter 3, we found that the advancements in text simplification research can have a positive impact on the medical domain. The research in the field of medicine is constantly developing and contains important information about drugs and treatments for various life threatening diseases. Although this information is accessible to the public, it is very complex in nature, thus making it difficult to understand. To address this problem, chapter 4 proposes an Automatic Text Simplification approach called “TESLEA”, which is capable of simplifying text related to the medical domain. The proposed approach employs a transformer-based model and leverages reinforcement learning to train the model in optimizing rewards that are tailored to text simplification. The proposed method outperformed previous baselines on Flesch-Kincaid scores (11.84) and achieved comparable performance with other baselines when measured using ROUGE-1 (0.39), ROUGE-2 (0.11), and SARI scores (0.40). The analysis of human annotated data revealed a percentage agreement of over 70% among human annotators when evaluated factors such as fluency, coherence, and adequacy. While having proposed an approach for simplifying medical text, this research also identifies potential avenues for future investigation, specifically the development of multilingual text simplification systems catering to diverse domains
Committee Members:
Dr. Vijay Mago (supervisor, committee chair), Dr. Garima Bajwa, Dr. Ameeta Agrawal (Maseeh College of Engineering and Computer Science, Portland State University)
Please contact grad.compsci@lakeheadu.ca for the Zoom link.
Everyone is welcome.