Computer Science Department Thesis Defense - Amirmohammad Shahbandegan
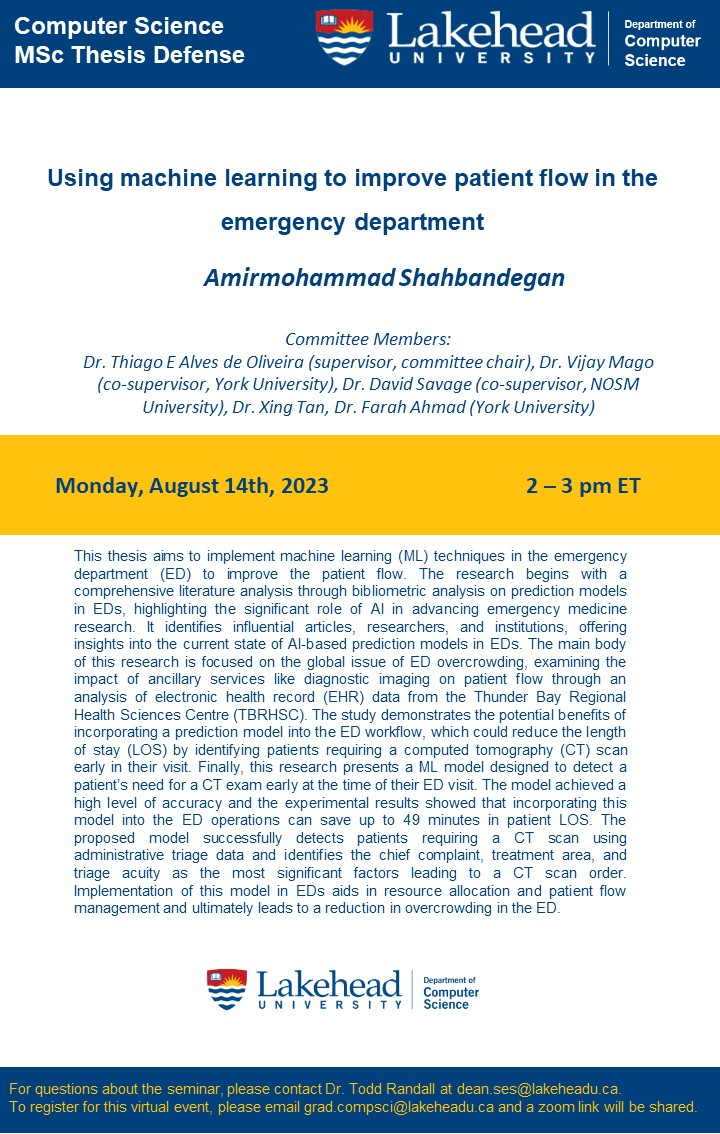
Please join the Computer Science Department for the upcoming thesis defense:
Presenter: Amirmohammad Shahbandegan
Thesis title: Using machine learning to improve patient flow in the emergency department
Abstract: This thesis aims to implement machine learning (ML) techniques in the emergency department (ED) to improve the patient flow. The research begins with a comprehensive literature analysis through bibliometric analysis on prediction models in EDs, highlighting the significant role of AI in advancing emergency medicine research. It identifies influential articles, researchers, and institutions, offering insights into the current state of AI-based prediction models in EDs. The main body of this research is focused on the global issue of ED overcrowding, examining the impact of ancillary services like diagnostic imaging on patient flow through an analysis of electronic health record (EHR) data from the Thunder Bay Regional Health Sciences Centre (TBRHSC). The study demonstrates the potential benefits of incorporating a prediction model into the ED workflow, which could reduce the length of stay (LOS) by identifying patients requiring a computed tomography (CT) scan early in their visit. Finally, this research presents a ML model designed to detect a patient’s need for a CT exam early at the time of their ED visit. The model achieved a high level of accuracy and the experimental results showed that incorporating this model into the ED operations can save up to 49 minutes in patient LOS. The proposed model successfully detects patients requiring a CT scan using administrative triage data and identifies the chief complaint, treatment area, and triage acuity as the most significant factors leading to a CT scan order. Implementation of this model in EDs aids in resource allocation and patient flow management and ultimately leads to a reduction in overcrowding in the ED.
Committee Members:
Dr. Thiago E Alves de Oliveira (supervisor, committee chair), Dr. Vijay Mago (co-supervisor, York University), Dr. David Savage (co-supervisor, NOSM University), Dr. Xing Tan, Dr. Farah Ahmad (York University)
Please contact grad.compsci@lakeheadu.ca for the Zoom link. Everyone is welcome.