Computer Science Department Thesis Defense - Sadman Sakib
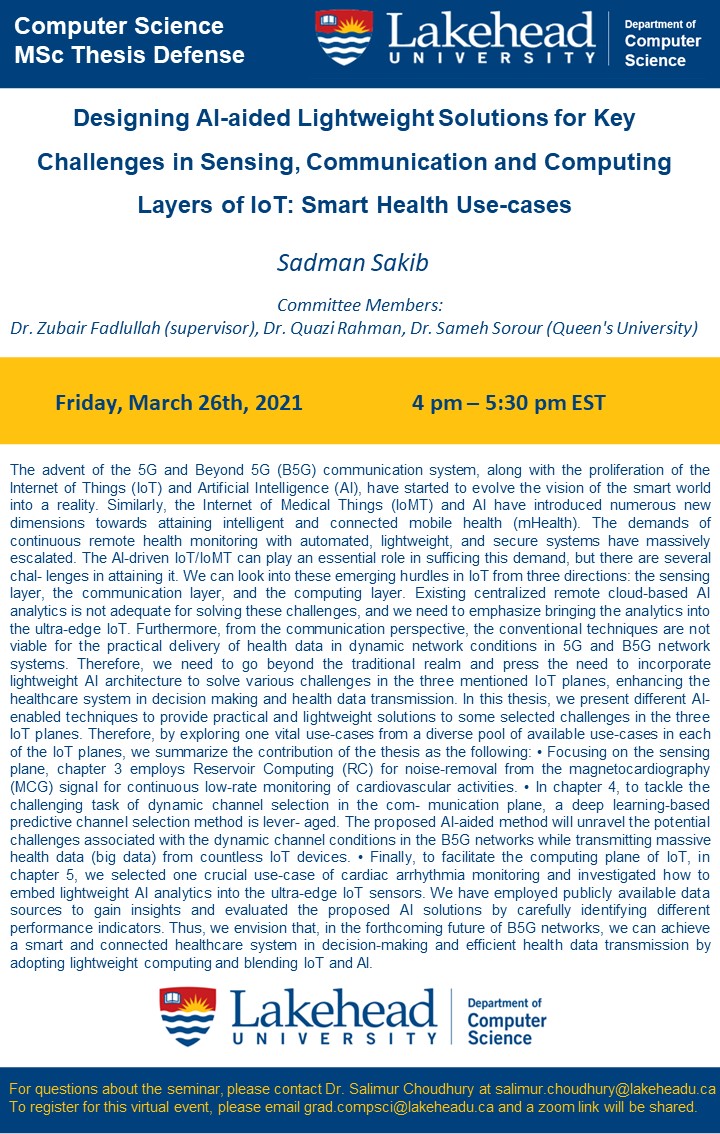
Please join the Computer Science Department for the upcoming thesis defense:
Presenter: Sadman Sakib
Thesis title: Designing AI-aided Lightweight Solutions for Key Challenges in Sensing, Communication and Computing Layers of IoT: Smart Health Use-cases
Abstract: The advent of the 5G and Beyond 5G (B5G) communication system, along with the proliferation of the Internet of Things (IoT) and Artificial Intelligence (AI), have started to evolve the vision of the smart world into a reality. Similarly, the Internet of Medical Things (IoMT) and AI have introduced numerous new dimensions towards attaining intelligent and connected mobile health (mHealth). The demands of continuous remote health monitoring with automated, lightweight, and secure systems have massively escalated. The AI-driven IoT/IoMT can play an essential role in sufficing this demand, but there are several chal- lenges in attaining it. We can look into these emerging hurdles in IoT from three directions: the sensing layer, the communication layer, and the computing layer. Existing centralized remote cloud-based AI analytics is not adequate for solving these challenges, and we need to emphasize bringing the analytics into the ultra-edge IoT. Furthermore, from the communication perspective, the conventional techniques are not viable for the practical delivery of health data in dynamic network conditions in 5G and B5G network systems. Therefore, we need to go beyond the traditional realm and press the need to incorporate lightweight AI architecture to solve various challenges in the three mentioned IoT planes, enhancing the healthcare system in decision making and health data transmission. In this thesis, we present different AI-enabled techniques to provide practical and lightweight solutions to some selected challenges in the three IoT planes. Therefore, by exploring one vital use-cases from a diverse pool of available use-cases in each of the IoT planes, we summarize the contribution of the thesis as the following: • Focusing on the sensing plane, chapter 3 employs Reservoir Computing (RC) for noise-removal from the magnetocardiography (MCG) signal for continuous low-rate monitoring of cardiovascular activities. • In chapter 4, to tackle the challenging task of dynamic channel selection in the communication plane, a deep learning-based predictive channel selection method is lever- aged. The proposed AI-aided method will unravel the potential challenges associated with the dynamic channel conditions in the B5G networks while transmitting massive health data (big data) from countless IoT devices. • Finally, to facilitate the computing plane of IoT, in chapter 5, we selected one crucial use-case of cardiac arrhythmia monitoring and investigated how to embed lightweight AI analytics into the ultra-edge IoT sensors. We have employed publicly available data sources to gain insights and evaluated the proposed AI solutions by carefully identifying different performance indicators. Thus, we envision that, in the forthcoming future of B5G networks, we can achieve a smart and connected healthcare system in decision-making and efficient health data transmission by adopting lightweight computing and blending IoT and AI.
Committee Members:
Dr. Zubair Fadlullah (supervisor, committee chair), Dr. Quazi Rahman, Dr. Sameh Sorour (Queen's University)
Please contact grad.compsci@lakeheadu.ca for the Zoom link.
Everyone is welcome.